Reinforcement Learning Meets Financial Risk: A Game-Changer for Decision Making?
- Anurag Kolla
- Oct 15, 2024
- 3 min read
Updated: Nov 19, 2024
Risk and reward are two sides of the same coin, especially in finance, but managing them has never been easy. Now, a cutting-edge approach is making waves by combining reinforcement learning (RL) with dynamic convex risk measures. In their recent paper, “Reinforcement Learning with Dynamic Convex Risk Measures,” Anthony Coache and Sebastian Jaimungal of the University of Toronto tackle one of the biggest challenges in risk-sensitive optimization and decision-making.
If terms like reinforcement learning and dynamic convex risk measures make your head spin, don't worry—we're here to break it down. Coache and Jaimungal’s work isn’t just for the finance nerds; it’s a forward-thinking take on how machines can help us manage uncertainty and make smarter decisions in risky environments.
So, What’s the Big Idea?
At its core, this paper blends two powerful concepts: RL, where machines learn from their environment by trial and error, and convex risk measures, which allow for more flexible and robust ways of handling risk over time. In simpler terms, it’s about teaching machines not just to maximize rewards (like profit) but also to be aware of potential downsides (like loss or volatility) along the way. The genius? They’ve done this without needing an explicit model of the environment, which is crucial in fields like finance where the future is unpredictable.
This isn’t your typical reinforcement learning approach where agents learn by sticking to what works most of the time (hello, “average performance”). Instead, the authors introduce dynamic convex risk measures, allowing the RL algorithm to account for worst-case scenarios—like a financial crash or sudden market disruption. This is what sets their work apart: it’s not just about finding a strategy that works most of the time; it’s about finding one that avoids disastrous losses when things go wrong.
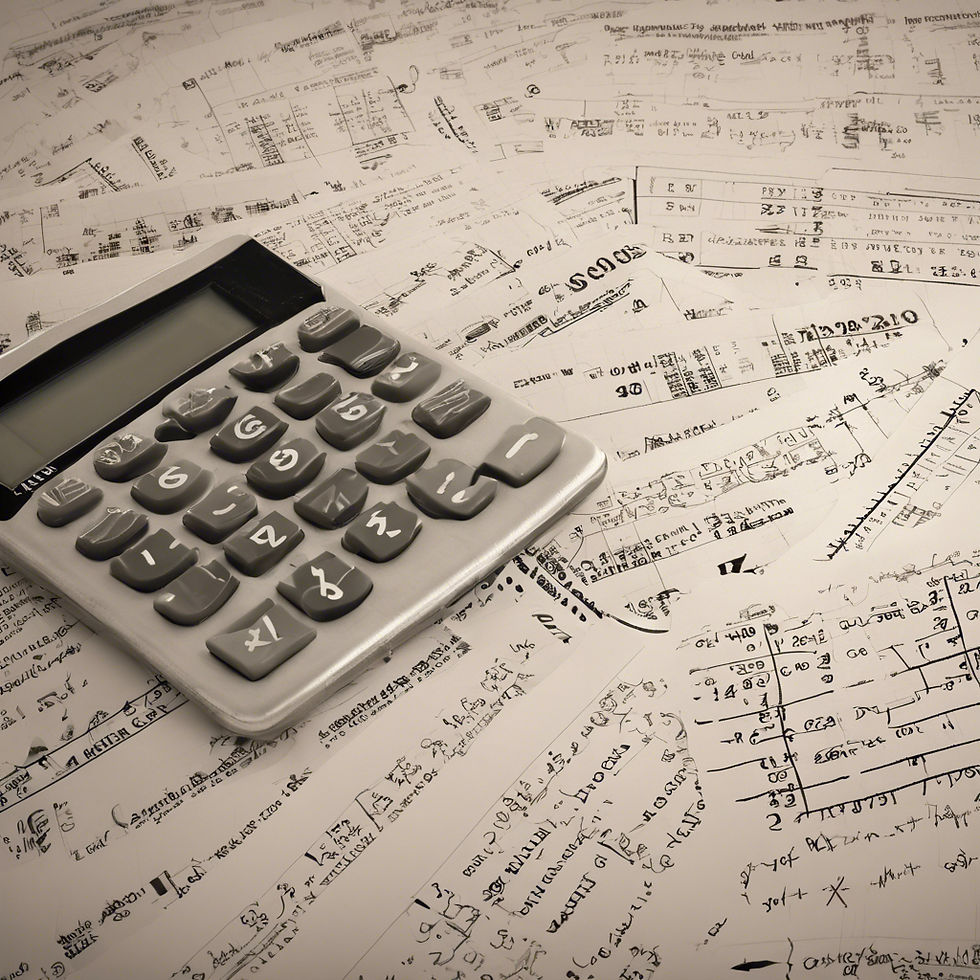
Why Does This Matter?
Let’s talk applications—because Coache and Jaimungal aren’t just theorizing in a vacuum. They’ve demonstrated the flexibility and power of their approach with real-world problems like:
Statistical arbitrage trading: Developing trading strategies that balance risk and reward in uncertain markets.
Financial hedging: Helping investors protect themselves from downside risk in volatile markets.
Robot control: Ensuring robots avoid obstacles in unpredictable environments, perfect for autonomous vehicles or drones.
These use cases highlight how RL combined with risk measures isn’t limited to finance—it could transform industries that deal with uncertainty, from autonomous vehicles to healthcare.
The Magic Behind the Math
What makes this paper a standout is the clever use of actor–critic algorithms (a type of RL where one network learns the strategy while another evaluates it) powered by neural networks. These algorithms are designed to continuously improve their risk-sensitive strategies through interactions with their environment. So whether it's optimizing a portfolio or controlling a robot, this approach can help find strategies that consistently minimize risk while maximizing gains.
Coache and Jaimungal’s approach is also time-consistent, meaning it avoids decisions today that could become risky tomorrow. This is huge in fields like finance, where what seems like a good decision in the short term could turn into a disaster long-term if risks aren’t carefully managed.
The Bottom Line
This paper may be rooted in complex math and algorithms, but its implications are far-reaching. As industries across the board look for ways to navigate uncertainty—whether it’s financial markets, supply chains, or autonomous systems—the methods proposed by Coache and Jaimungal could help them do so with greater confidence.
The next time you see a self-driving car navigating a tricky road, or hear about an algorithm managing a billion-dollar portfolio, remember: it’s breakthroughs like this that are helping us not just aim for the stars but also avoid the pitfalls along the way. In a world increasingly driven by data, this blend of machine learning and risk management might just be the key to unlocking smarter, safer decision-making.
Bibliography:
Coache, Anthony, and Sebastian Jaimungal. ‘Reinforcement Learning with Dynamic Convex Risk Measures’. CoRR, vol. abs/2112.13414, 2021, https://arxiv.org/abs/2112.13414.
Comentarios